Abstract:
The traditional geophysical modeling workflows provide high-quality predictions but are often computationally demanding. For real-time applications, such as real-time well-placement optimization (geosteering), it is important to get approximate modeling results instantaneously. In this communication we demonstrate how different deep learning models can be used to (a) create a subsurface model prediction and (b) electro-magnetic log approximation. These models are integrated into a Bayesian workflow which enables a probabilistic prediction of ahead-of-bit, which in turn can aid operational decision-making.
For (a) we propose to use Generative Adversarial Network (GAN) trained on a realistic geological configuration for the geomodel prediction. This method creates a mathematically-sound parametrization of subsurface which uses few implicitly defined geological parameters.
Our forward deep neural network (FDNN) model (b) approximates the responses of all 22 logs of extra-deep EM tool sent in real time. This gives a look-around capability of up to 30 meters. FDNN has 50 times faster performance compare to a commercial high-fidelity simulator.
Biography:
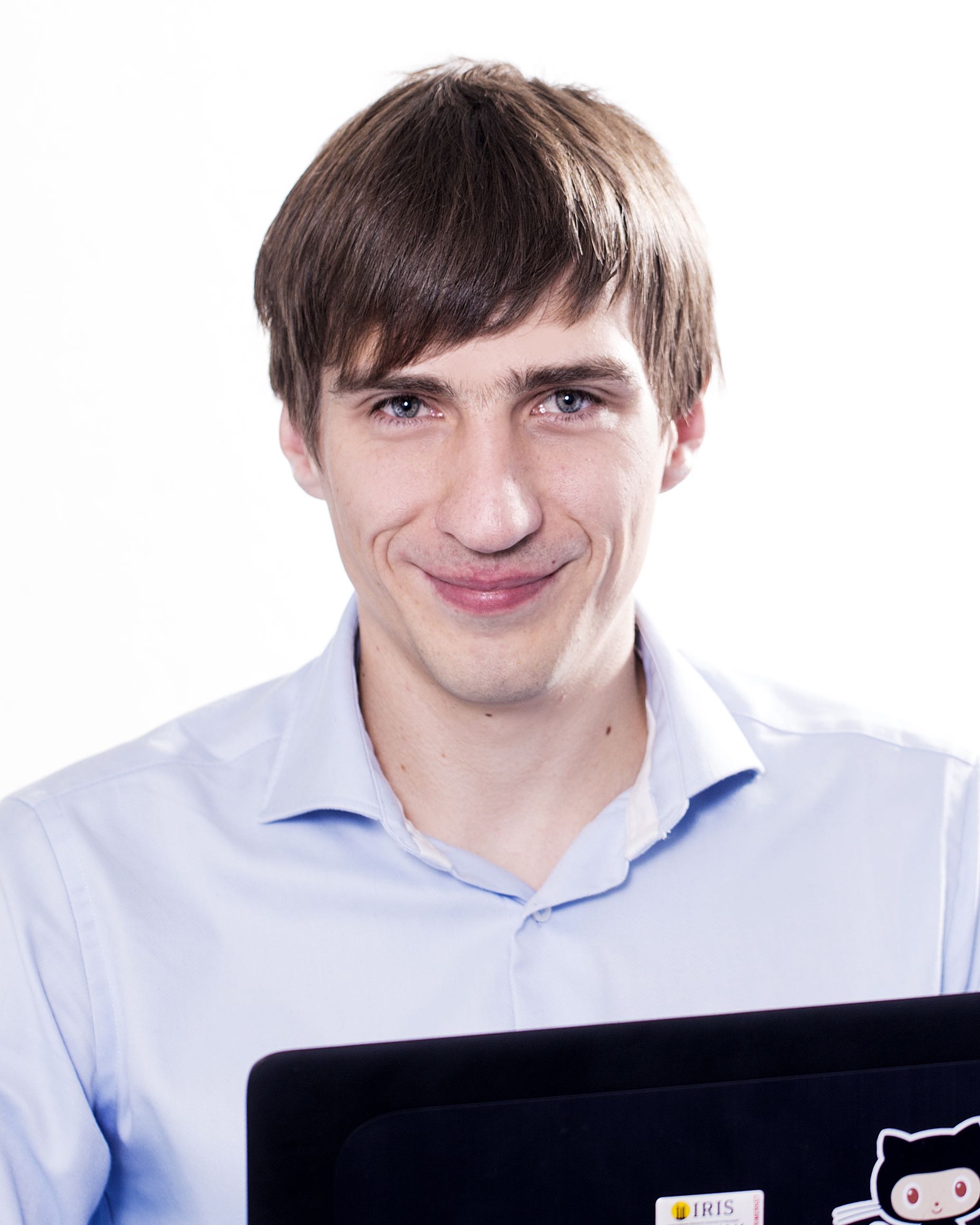
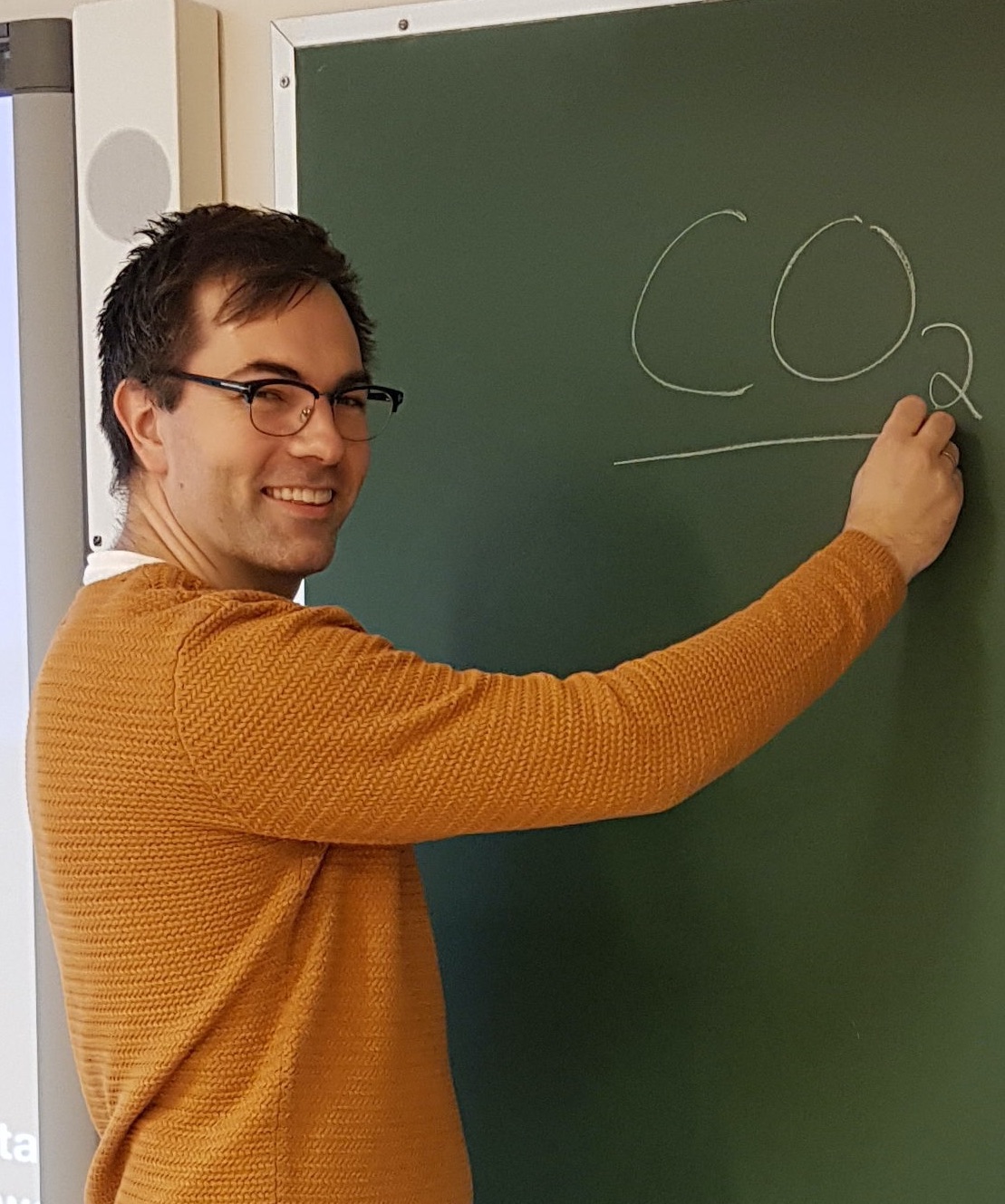