The presentation “Real-time Reservoir Fluid Identification from Mud Gas” will be given by Dr. Tao Yang, from Equinor.
Abstract:
Reservoir fluid sampling or downhole fluid analysis are the main technologies to acquire reservoir fluid properties. However, many real-time well decisions are made before or without wireline logging. Logging while drilling (especially density-neutron separation) is the primary tool to identify reservoir oil or gas. However, the fluid typing accuracy from density-neutron separation is not satisfied, and any misinterpretation will lead to large consequences regarding well placement and completion decisions. Therefore, there is a strong business need to provide accurate reservoir oil or gas identification while drilling.
Mud gas data is available near real-time for all the wells. The data is mainly used by drilling as a safety measure and by geochemistry to study the petroleum systems for post well use. Our digital innovation identified a strong relationship between mud gas data and reservoir fluid properties using a machine learning algorithm. The new method turned the underused mud gas data into real-time fluid identification, which is highly valued for real-time well decisions. Field cases are given to demonstrate that the new technology created significant business values.
The success of the technology shows digital innovation solved a long-lasting technical challenge in the oil industry. The in-expensive mud gas data provide accurate and continuous reservoir fluid property prediction in the drilling phase. The new method significantly improves the real-time well decisions. The innovation also reshapes the mud gas service in the industry as a real-time service for much broader user groups beyond drilling and geochemistry, especially for well placement, completion, and production.
Biography:
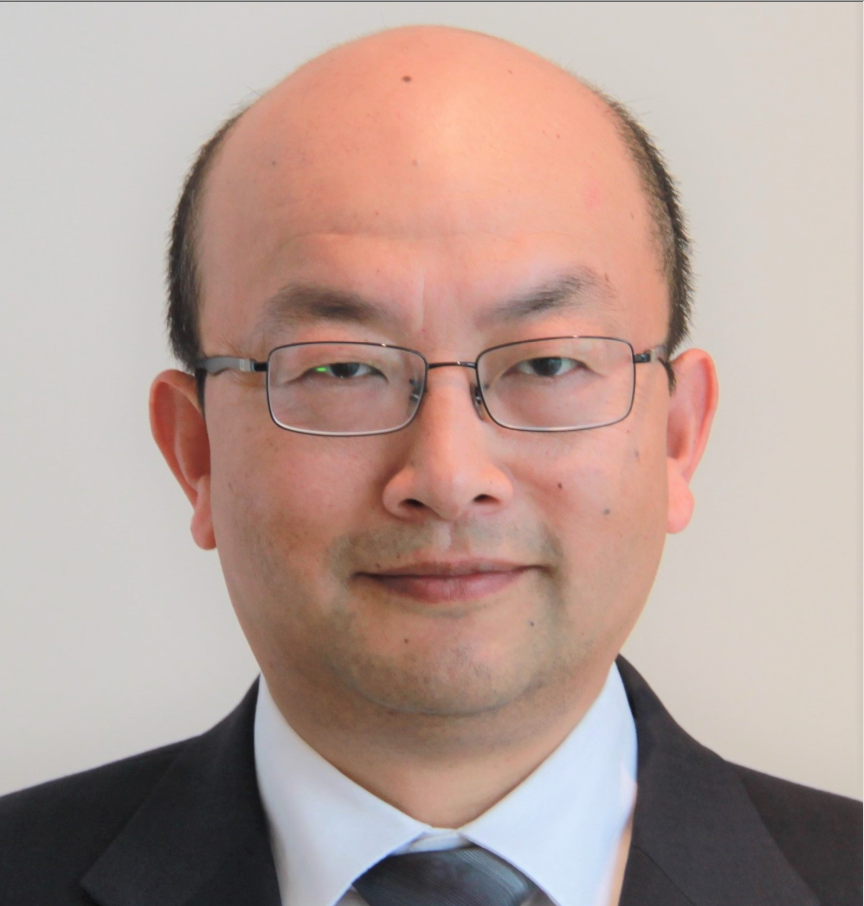